Towards Robust Blind Face Restoration with
Codebook Lookup TransFormer
Video Demo (Download full video | short video)
Materials
Abstract
Blind face restoration is a highly ill-posed problem that often requires auxiliary guidance to 1) improve the mapping from degraded inputs to desired outputs, or 2) complement high-quality details lost in the inputs. In this paper, we demonstrate that the learned discrete codebook prior in a small proxy space largely reduces the uncertainty and ambiguity of restoration mapping by casting face restoration as a code prediction task, it meanwhile provides rich visual atoms for generating high-quality faces. Under this paradigm, we propose a Transformer-based prediction network, named CodeFormer, to model global composition and context of the low-quality faces for code prediction, enabling the discovery of natural faces that closely approximate the target faces even when the inputs are severely degraded. To enhance the adaptiveness for different degradation, we also propose a controllable feature transformation module that allows a flexible trade-off between fidelity and quality. Thanks to the expressive codebook prior and global modeling, CodeFormer outperforms the state-of-the-arts in both quality and fidelity, showing superior robustness to degradation. Extensive experimental results on synthetic and real-world datasets verify the effectiveness of our method.
Method
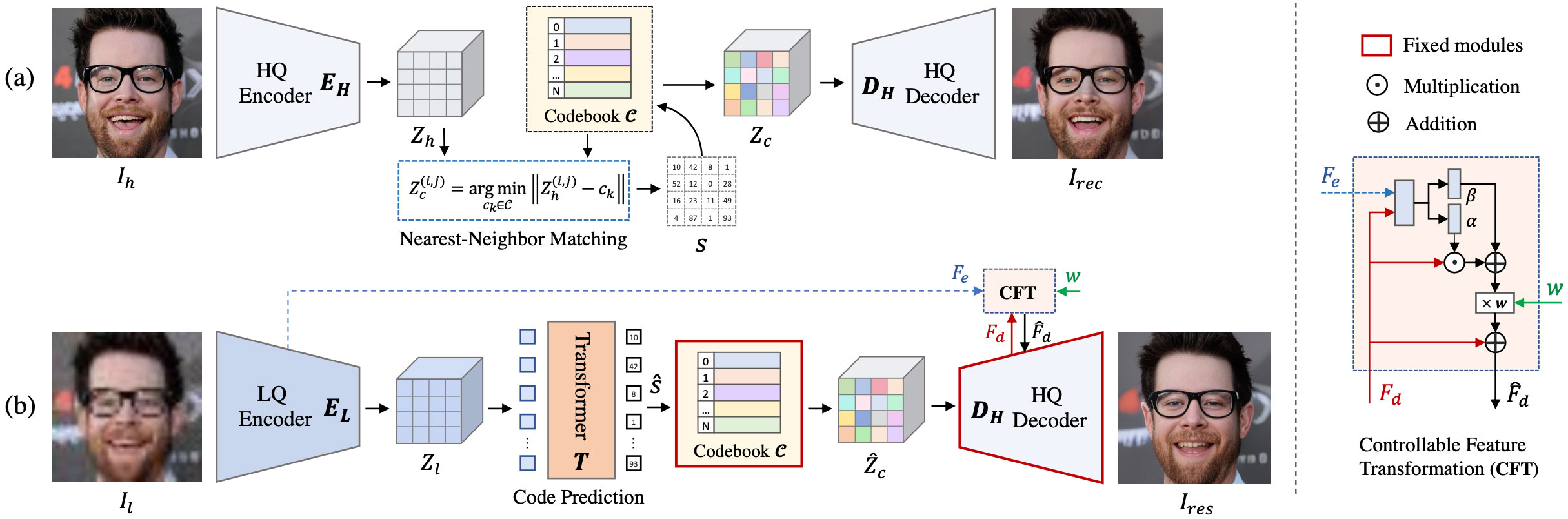
Overview of CodeFormer
(a) We first learn a discrete codebook and a decoder to store high-quality visual parts of face images via self-reconstruction learning. (b) With fixed codebook and decoder, we then introduce a Transformer module for code sequence prediction, modeling the global face composition of low- quality inputs. Besides, a controllable feature transformation module is used to control the information flow from LQ encoder to decoder. Note that this connection is optional, which can be disabled to avoid adverse effects when inputs are severely degraded, and one can adjust a scalar weight w to trade between quality and fidelity.
Trade-off between Quality and Fidelity
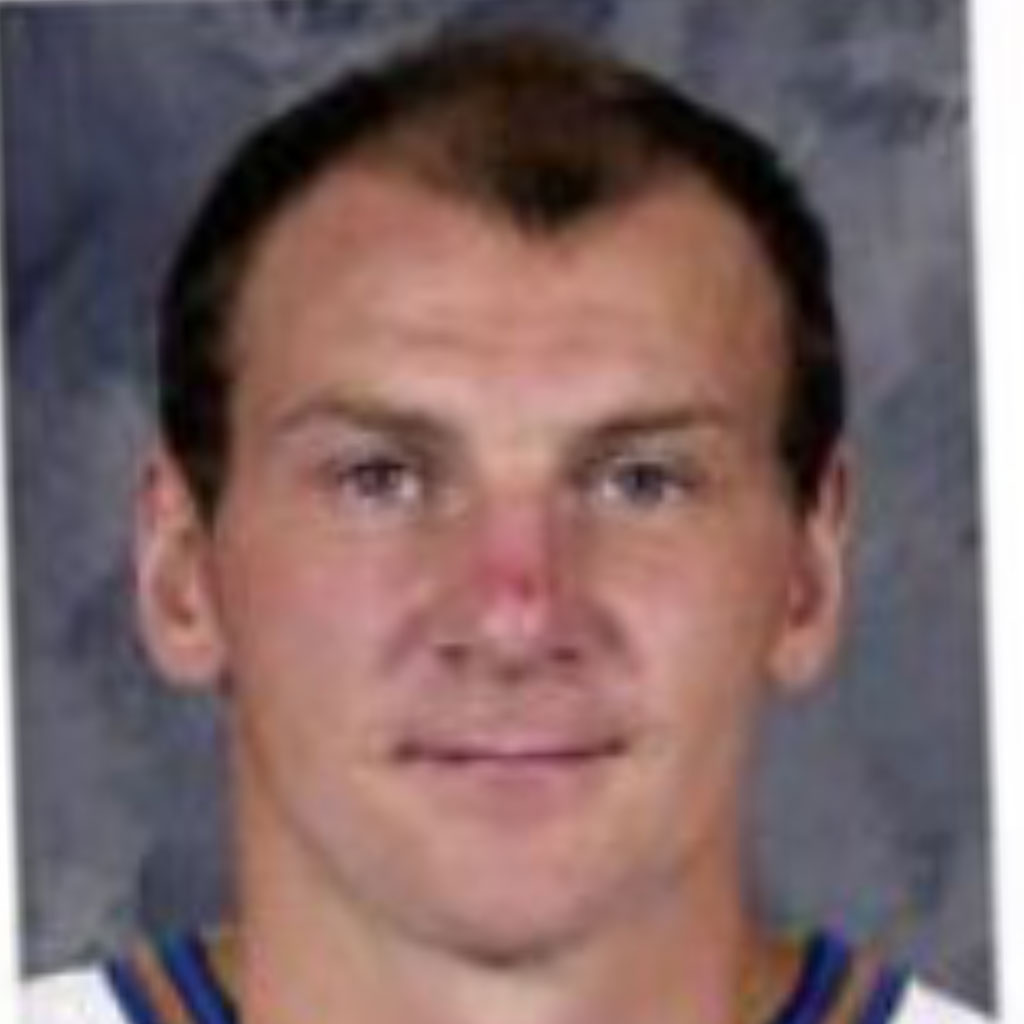
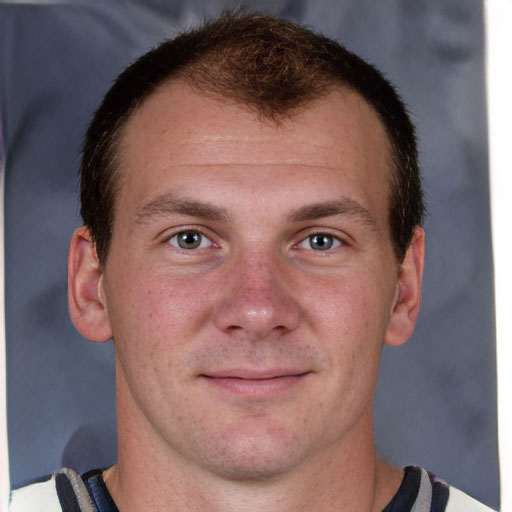
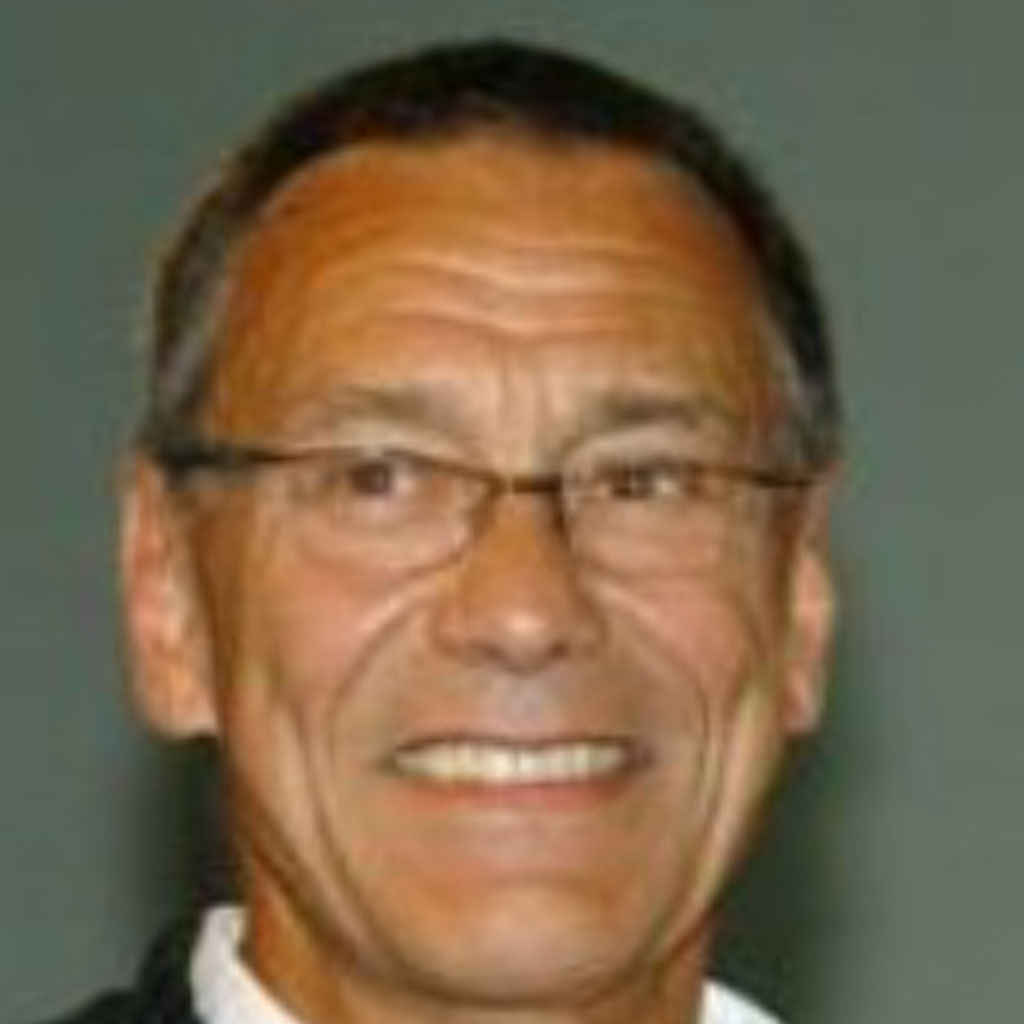
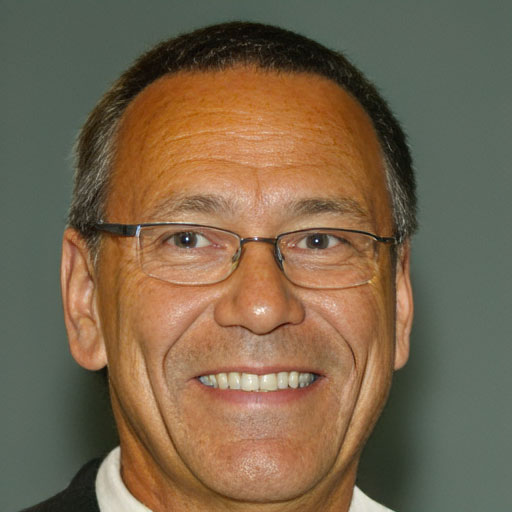
Controllable Transitions between Image Quality and Fidelity.
(A smaller w tends to produce a high-quality result while a larger w improves the fidelity.)Results
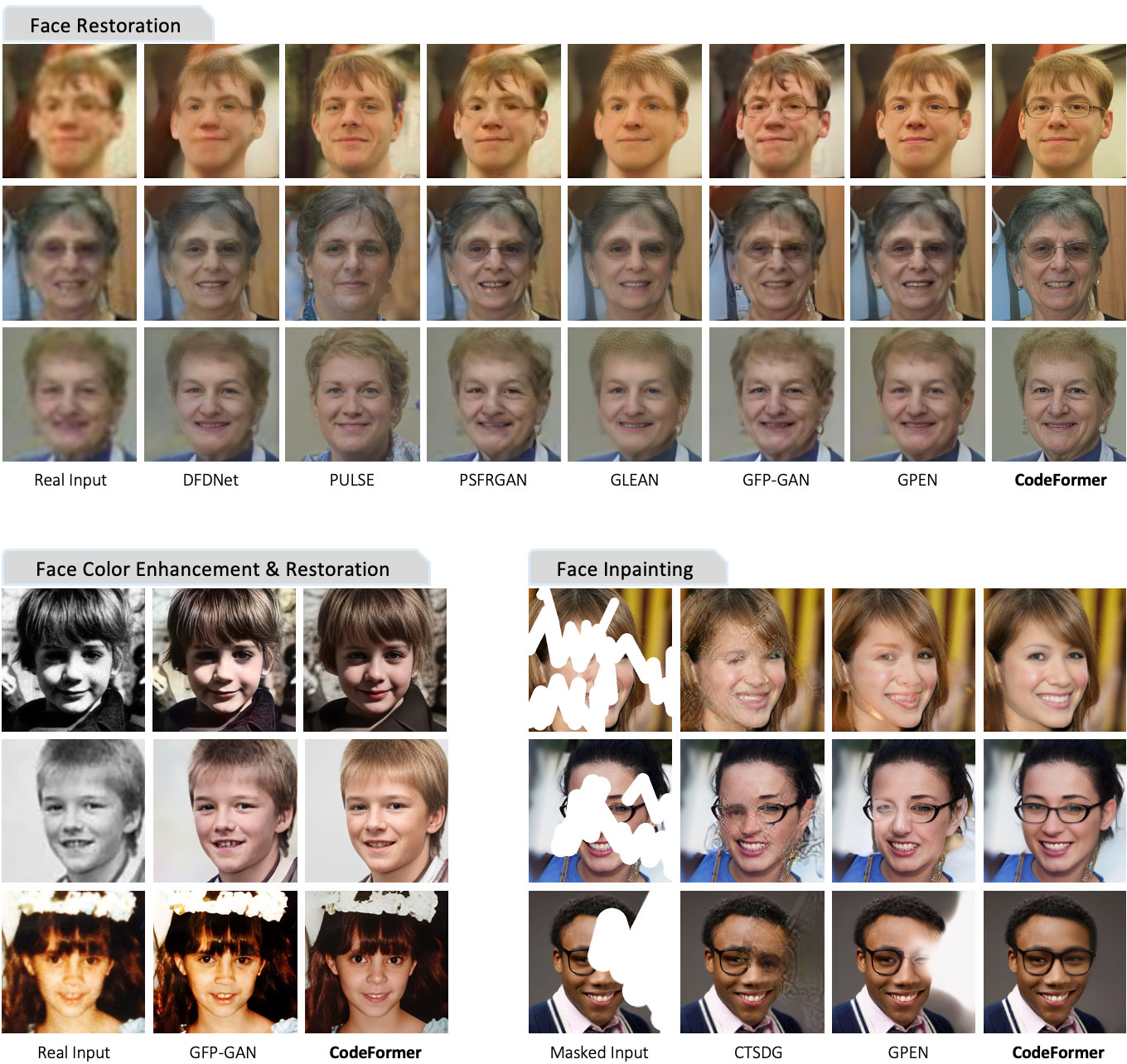
Citation
If you find our dataset and paper useful for your research, please consider citing our work:
@inproceedings{zhou2022codeformer, author = {Zhou, Shangchen and Chan, Kelvin C.K. and Li, Chongyi and Loy, Chen Change}, title = {Towards Robust Blind Face Restoration with Codebook Lookup TransFormer}, booktitle = {NeurIPS}, year = {2022} }
Contact
If you have any question, please contact us at shangchenzhou@gmail.com.