LEDNet: Joint Low-light Enhancement and Deblurring in the Dark
![]() |
![]() |
![]() |
![]() |
![]() |
![]() |
Visual results on real-world night blurry images (MouseOut: input, MouseOver: result)
Visual results on real-world night blurry videos
Abstract
Night photography typically suffers from both low light and blurring issues due to the dim environment and the common use of long exposure. While existing light enhancement and deblurring methods could deal with each problem individually, a cascade of such methods cannot work harmoniously to cope well with joint degradation of visibility and textures. Training an end-to-end network is also infeasible as no paired data is available to characterize the coexistence of low light and blurs. We address the problem by introducing a novel data synthesis pipeline that models realistic low-light blurring degradations. With the pipeline, we present the first large-scale dataset for joint low-light enhancement and deblurring. The dataset, LOL-Blur, contains 12,000 low-blur/normal-sharp pairs with diverse darkness and motion blurs in different scenarios. We further present an effective network, named LEDNet, to perform joint low-light enhancement and deblurring. Our network is unique as it is specially designed to consider the synergy between the two inter-connected tasks. Both the proposed dataset and network provide a foundation for this challenging joint task. Extensive experiments demonstrate the effectiveness of our method on both synthetic and real-world datasets.
Dataset
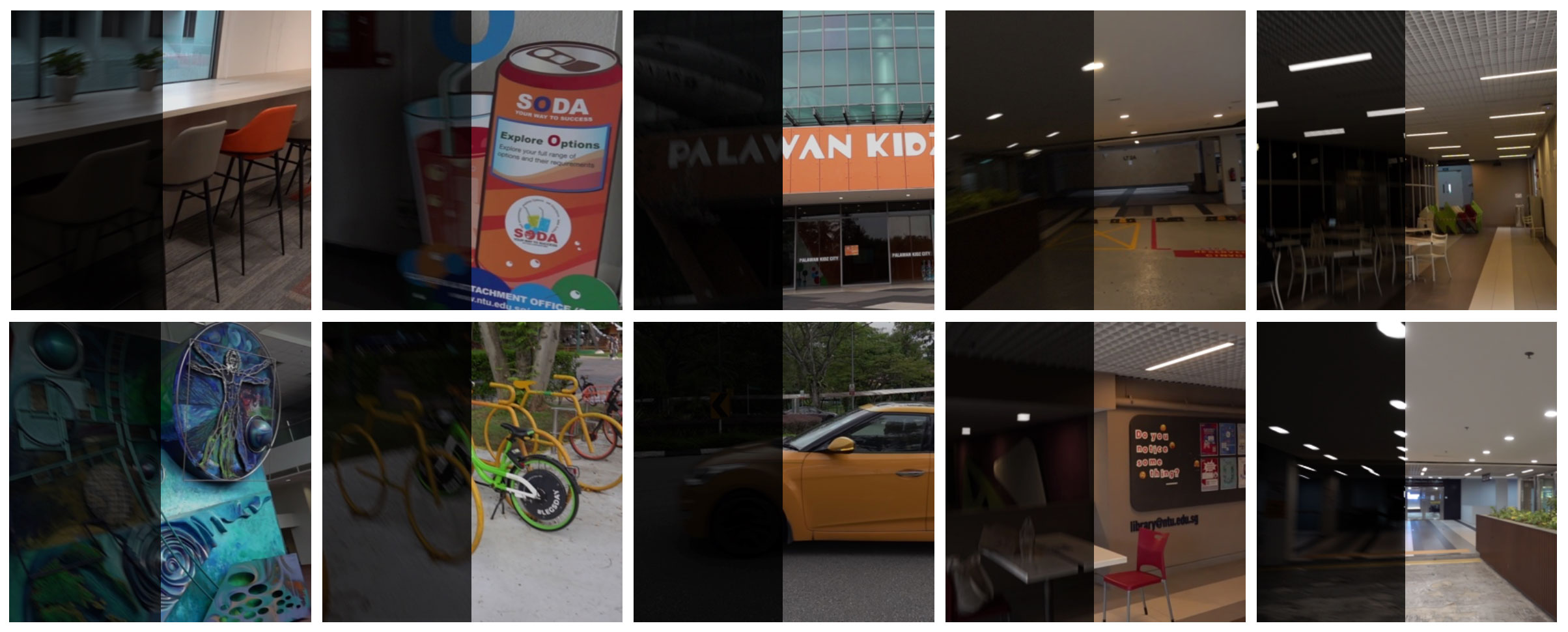
Examples in LOL-Blur dataset, showing diverse darkness and motion blurs in dark dynamic scenes. The dataset also provides realistic blurs in the simulated regions, like light streaks shown in the last two columns.
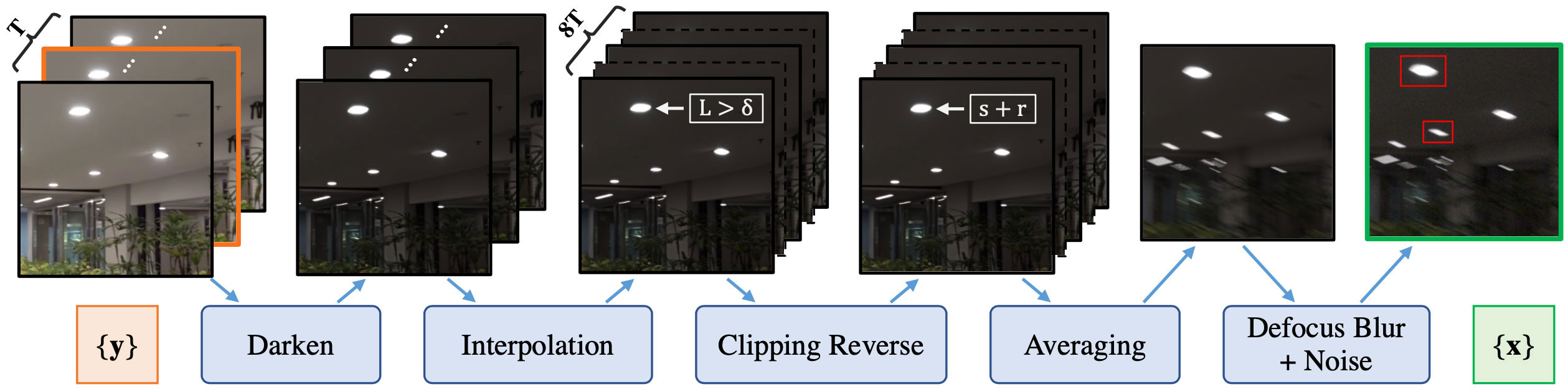
We provide a data synthesis pipeline that models low-light blur degradation realistically, hence allowing us to generate a large-scale dataset (LOL-Blur) data for this joint task. The pipeline contains five main steps, as shown in the above figure. Based on this synthesis pipeline, we construct a large-scale dataset LOL-Blur, consisting of a total of 10,200 pairs {x,y} for training and 1,800 pairs {x,y} for testing.
Method
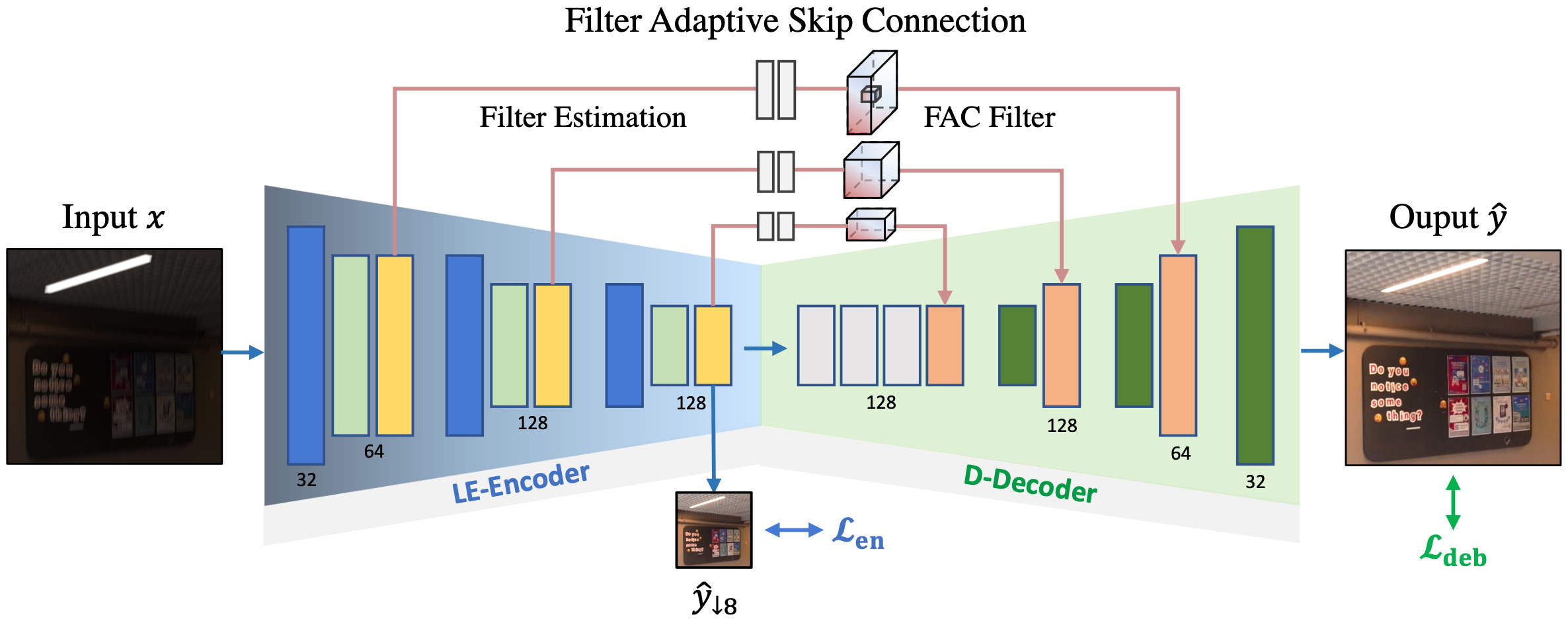
It contains an Encoder for Light Enhancement, LE-Encoder, and a Decoder for Deblurring, D-Decoder. They are connected by three Filter Adaptive Skip Connections (FASC). The PPM and the proposed CurveNLU layers are inserted in LE-Encoder, making light enhancement more stable and powerful. LEDNet applies spatially-adaptive transformation to D-Decoder using filters generated by FASC from enhanced features. CurveNLU and FASC enable LEDNet to perform spatially-varying feature transformation for both intensity enhancement and blur removal.
Results
Citation
If you find our dataset and paper useful for your research, please consider citing our work:
@article{zhou2022lednet, author = {Zhou, Shangchen and Li, Chongyi and Loy, Chen Change}, title = {LEDNet: Joint Low-light Enhancement and Deblurring in the Dark}, journal={arXiv preprint arXiv:2202.03373}, year = {2022} }
Contact
If you have any question, please contact us via shangchenzhou@gmail.com.